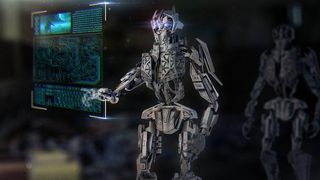
(Image credit: Pixabay.com © Computerizer CCO Public Domain)
- Robots war to study from every varied, and count on human instruction
- Novel evaluate from UC Berkeley reveals that the technique can be automated
- This would assign away with the struggles of manually practising robots
Regardless of robots being extra and additional built-in into real-world environments, one amongst the critical challenges in robotics evaluate is making sure the devices can adapt to composed projects and environments efficiently.
Traditionally, practising to master direct skills requires super amounts of info and the truth is knowledgeable practising for every robotic mannequin – nonetheless to beat these obstacles, researchers for the time being are focusing on increasing computational frameworks that allow the transfer of skills all the blueprint in which via varied robots.
A brand composed construction in robotics comes from researchers at UC Berkeley, who personal equipped RoVi-Aug – a framework designed to prolong robotic info and facilitate skill transfer.
The keep of skill transfer between robots
To ease the practising process in robotics, there is a can personal to be in a self-discipline to transfer discovered skills from one robotic to at least one other even supposing these robots personal varied hardware and make. This capacity would set it less complicated to deploy robots in a wide vary of applications with out having to retrain every from scratch.
Nonetheless, in many newest robotics datasets there is an uneven distribution of scenes and demonstrations. Some robots, comparable to the Franka and xArm manipulators, dominate these datasets, making it extra great to generalize discovered skills to varied robots.
To address the obstacles of present datasets and objects, the UC Berkeley team developed the RoVi-Aug framework which uses say-of-the-artwork diffusion objects to prolong robotic info. The framework works by producing synthetic visual demonstrations that adjust in both robotic kind and digicam angles. This permits researchers to prepare robots on a wider vary of demonstrations, enabling extra efficient skill transfer.
The framework contains two key elements: the robotic augmentation (Ro-Aug) module and the standpoint augmentation (Vi-Aug) module.
The Ro-Aug module generates demonstrations intriguing varied robotic systems, whereas the Vi-Aug module creates demonstrations captured from diversified digicam angles. Collectively, these modules present a richer and additional diverse dataset for practising robots, serving to to bridge the gap between varied objects and projects.
“The success of novel machine discovering out systems, seriously generative objects, demonstrates impressive generalizability and motivated robotics researchers to search out carry out same generalizability in robotics,” Lawrence Chen (Ph.D. Candidate, AUTOLab, EECS & IEOR, BAIR, UC Berkeley) and Chenfeng Xu (Ph.D. Candidate, Pallas Lab & MSC Lab, EECS & ME, BAIR, UC Berkeley), suggested Tech Xplore.